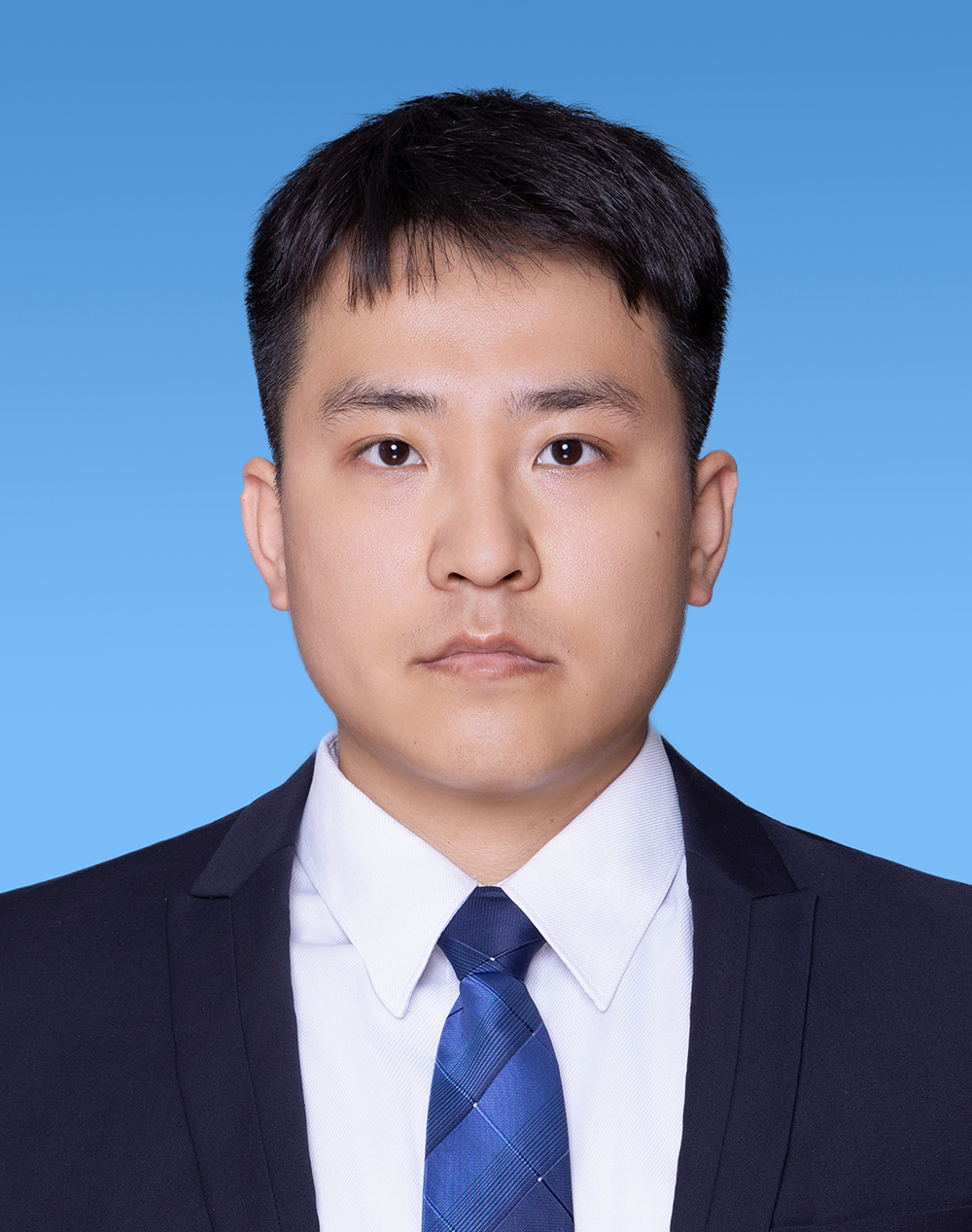
李龙飞,男,博士,讲师,河南工业大学青年教师。
现主要从事人工智能、数据挖掘、医学影像分析、医工交叉融合等研究工作,具体方向包括计算机视觉、深度学习、影像组学和医疗大数据分析等。在国际知名期刊和会议上发表学术论文20余篇;授权/受理国家发明专利2余项。主持科研启动项目1项,参与多项国家级项目。
通讯地址:河南省郑州市莲花街100号
电子邮箱:lfli@haut.edu.cn
教育与工作经历
2012.09-2016.06 郑州大学 软件工程 学士学位
2016.09-2023.07 郑州大学 软件工程 博士学位
2017.10-2019.09 中国科学院自动化研究所 联培
2020.10-2022.01 中国科学院深圳先进技术研究院 联培
2023.09-至今 河南工业大学 讲师
实验室和科研团队简介
所在团队于致力于人工智能、计算机视觉及其在中医诊断数据分析、疾病辅助诊断建模等方面的应用研究。通过利用现代技术采集的舌象、脉象、病症等反映多种慢性疾病状态的多模态数据,基于大数据、人工智能等技术进行分析,挖掘患者的中医表征与病症发展变化的复杂映射关系,实现针对中医的辅助诊断。
研究领域与方向
1.中医多模态数据挖掘。通过分析和整合来自中医不同来源的多种数据类型(如文本、图像、声音等),以揭示中医学中的复杂模式和关联。这一领域利用现代数据挖掘技术,如机器学习和深度学习,对中医诊断、治疗、药物配比等方面的大量数据进行分析,以提高中医临床决策的准确性和效率。研究方向包括但不限于中药复方的效果预测、疾病诊断模型的建立、以及中医知识的发现和验证等。通过这些研究,旨在推动中医学的现代化和国际化进程。
2. 辅助诊断模型构建。研究领域专注于开发和应用先进的计算技术,以辅助中医的诊断过程。这些模型通过分析患者的临床数据、舌象、脉象等传统中医诊断信息,结合现代机器学习和人工智能技术,旨在提高诊断的准确性和效率。研究方向包括但不限于深度学习在中医图像识别中的应用、自然语言处理技术用于解析中医文本、以及大数据分析用于中医疾病模式识别等。这些研究有望极大地增强中医诊断的客观性和科学性,为患者提供更为精准和个性化的治疗方案。
研究成果
(一)主持的科研项目
2024/3-2027/3,主持校级科研启动项目, “脑肿瘤影像辅助诊断方法研究”,在研;
(二)代表著作与论文
代表性论文:
[1] Li Longfei, et al. Universal multi-factor feature selection method for radiomics-based brain tumor classification[J]. Computers in Biology and Medicine, 2023: 107122.(影响因子:7.7,中科院一区)
[2] Li Longfei, et al. Radiomic analysis of multiparametric magnetic resonance imaging for differentiating skull base chordoma and chondrosarcoma[J]. European journal of radiology, 2019, 118: 81-87.(影响因子:4.531,中科院二区)
[3] Li Longfei, et al. A Non-invasive Radiomic Method Using 18F-FDG PET Predicts Isocitrate Dehydrogenase Genotype and Prognosis in Patients with Glioma[J]. Frontiers in oncology, 2019, 9: 1183.
(影响因子:6.244,中科院二区)
[4] Li Longfei, et al. Radiomic biomarker extracted from PI-RADS 3 patients support more efficient and robust prostate cancer diagnosis: a multi-center study. Abstract, ISMRM, 2022, digital poster.
[5] Wang Y, Li Longfei, et al. Expert knowledge guided manifold representation learning for magnetic resonance imaging-based glioma grading[J]. Biomedical Signal Processing and Control, 2023, 85: 104876.
(影响因子:5.1,中科院二区,共同第一作者)
[6] Fan Y, Liu Z, Hou B, Li Longfei, et al. Development and validation of an MRI-based radiomic signature for the preoperative prediction of treatment response in patients with invasive functional pituitary adenoma[J]. European Journal of Radiology, 2019, 121: 108647.(影响因子:4.531,中科院二区,共同第一作者)
[7] Guohua Zhao, Panpan Man, Jie Bai, Longfei Li, et al. “AI-Powered Radiomics Algorithm Based on Slice Pooling for the Glioma Grading.” IEEE Transactions on Industrial Informatics 18(8): 5383-5393 (2022) (影响因子:12.3,中科院一区Top)
[8] Liu, Zhenyu, Zhuolin Li, Jinrong Qu, Renzhi Zhang, Xuezhi Zhou, Longfei Li, Kai Sun, et al. "Radiomics of multiparametric MRI for pretreatment prediction of pathologic complete response to neoadjuvant chemotherapy in breast cancer: a multicenter study." Clinical Cancer Research 25, no. 12 (2019): 3538-3547. (影响因子:13.801,中科院一区Top)
[9] Liu, Zhenyu, Shuo Wang, Jingwei Wei, Di Dong, Cheng Fang, Xuezhi Zhou, Kai Sun, Longfei Li, Bo Li, Meiyun Wang, and Jie Tian. "The applications of radiomics in precision diagnosis and treatment of oncology: opportunities and challenges." Theranostics 9, no. 5 (2019): 1303. (影响因子:12.4,中科院一区Top)
[10] Qingxia Wu, Kuan Yao, Zhenyu Liu, Longfei Li, Xin Zhao, Shuo Wang, Honglei Shang, Yusong Lin, Zejun Wen, Xiaoan Zhang, Jie Tian, Meiyun Wang. Radiomics analysis of placenta on T2WI facilitates prediction of postpartum haemorrhage: A multicentre study[J]. EBioMedicine, 2019, 50: 355-365. (影响因子:11.2,中科院一区Top)